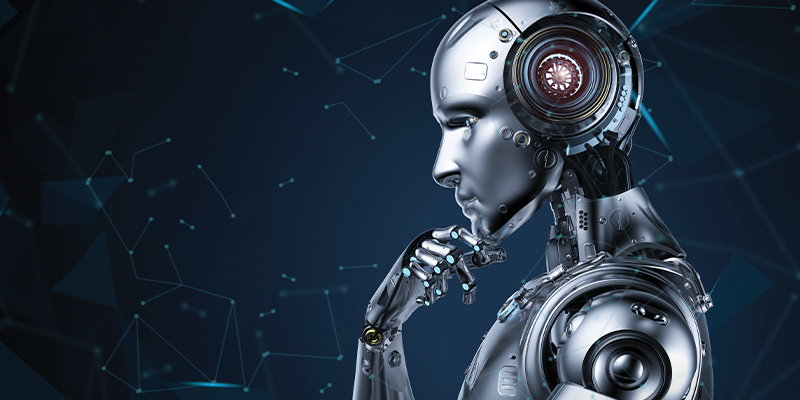
In today’s era of accelerating innovation, artificial intelligence (AI), driven by advances in machine learning, has become a ubiquitous presence in our lives. It seems like every time we turn on the news or browse the web, there’s a new AI-related story in the headlines.
So, what does AI bring to the field of hematologic oncology? The answer lies in its potential to revolutionize the way we approach the diagnosis, treatment, and understanding of blood-related cancers.1,2
“I think that we’ll see it eventually at every stage of the patient’s journey, starting from diagnosis to treatment to relapse to quality of life after the treatment or survivorship,” said Roni Shouval, MD, PhD, a physician-scientist at Memorial Sloan Kettering Cancer Center in New York City. “At every time point, there may be an application for AI.”
Dr. Shouval’s choice of the words “eventually” and “may” reflects the hesitancy that is typical among researchers in the field. It is clear that the full potential of AI in hematologic oncology has yet to be realized, despite all the hype.
“When it comes to machine learning and AI, you have to separate the hype from where the value is—because there is value,” said Aziz Nazha, MD, Global Head of Incyte’s AI Innovations Institute. “But I think sometimes the hype overtakes the value. Once you focus too much on the hype, then it becomes a problem when the expectation of the technology is not getting realized, and then people become skeptical.”
Making Inroads in the Field of Hematology
As AI continues to make inroads in the field of hematology, its usefulness is most evident in diagnostics, where it serves as a complementary tool rather than a replacement for physicians. The technology has the ability to significantly enhance both the speed and accuracy of diagnostics, and even to distinguish rare blood cancers that pose challenges to hematopathologists.3
“Hematology in general, especially malignant hematology, is such a unique field where you have several technologies,” Dr. Nazha observed. “You have imaging flow cytometry and clinical and laboratory data, so you have multimodality data to make the diagnosis, and that’s a great use case for machine learning.”
The interpretation of pathology assays, including bone marrow smears and peripheral blood smears, is one of the first direct implementations of the technology in the field, according to Dr. Shouval.
“The concept is that you can help these labor-intensive, experience-based tasks by AI augmenting the ability of the pathologist to accurately perform these tests and do it at large scale,” Dr. Shouval said. “The computers don’t get tired, and you can at least try to program them to have less bias.”
Another area of application is in the diagnosis of myelodysplastic syndromes (MDS), which are particularly challenging due to their close resemblance to other myeloid malignancies. In some instances, the only definitive differentiation can be achieved through a bone marrow biopsy, Dr. Nazha said.
To overcome this diagnostic challenge, Dr. Nazha and colleagues developed a machine learning model capable of distinguishing MDS from other myeloid diseases solely by analyzing complete blood counts (CBC), differentials, and next-generation, targeted, deep-sequencing data.4
This type of CBC model is only one of the many potential approaches to diagnosing MDS, Dr. Nazha said.
“For example, you can use computer vision to look at cells in bone marrow biopsy and say these are malignant or dysplastic cells,” he noted. “Or you could use the clinical lab data in the structure notes from the pathology report to build a model to predict the disease diagnosis.”
Diagnosis is just the first in a chain of potential clinical applications for AI. Other applications include prognosis and determining the next step in a patient’s treatment.
“The right treatment at the right time can sometimes be challenging in cancer in general and certainly in hematologic malignancies,” Dr. Nazha said.
Clinicians might use AI to decide whether to proceed with a transplant or to determine the appropriate intensity of chemotherapy.
“You could use machine learning to try to build models to predict response or resistance to chemo[therapy] and to understand the variables that impact that response and resistance,” Dr. Nazha said.
However, Dr. Shouval cautioned that, at present, there is not yet much actual clinical utility for models that try to predict outcomes and make treatment or clinical trial recommendations.
”One, many of these models have not been validated externally, so you can’t really run them on your own data safely,” Dr. Shouval said. “Second, a lot of them still suffer from relatively low predictive performance. And third, because there’s so much variation in the different populations that we treat, it’s sometimes hard to extrapolate one model from one population to the other because of this variance. That’s why in order to really incorporate these models, we need good validation sets and collaboration.”
Far From Perfect
While AI holds tremendous promise, it’s currently far from a panacea in the field. There are hurdles to overcome, such as data privacy concerns, the need for robust validation of AI models, a lack of qualified personnel, and the requirement for large and diverse datasets to train these models effectively. Moreover, integrating AI seamlessly into clinical practice and ensuring that it consistently benefits patients are ongoing challenges.
“I think one of the challenges for running or developing robust prediction models or diagnostic models in medicine is access to large datasets,” said Dr. Shouval. “When you look at the algorithms that are currently used, the deep-learning algorithms, the large language models [LLMs], the ones that are used by ChatGPT and others, these need billions of samples to learn from.”
Dr. Shouval believes that, given the challenges related to accessing large datasets and the limited representation of patients in pharmaceutical companies’ clinical trials, the future of AI and machine learning in hematologic oncology lies in academia and collaborative centers.
“It’s a challenge for pharmaceutical companies because they don’t have the full spectrum of patients in their clinical trials; it’s a selective group that’s not reflective of what’s out there in the real world,” he said. “To actually train machine learning models or AI models, you need to learn from variation. So having larger datasets that are not siloed at one institution or with one company is a great advantage.”
The field also has a talent challenge, which further hinders the progress of the technology, according to Dr. Nazha.
“We as physicians are not trained to understand machine learning and AI,” he said. “The data scientists, on the other hand, approach the data in health care similar to banking and other types of data, which we all know is completely different. This data is messy, [there are] a lot of missing data. Sometimes you have a smaller patient population. So, there are a lot of intrinsic challenges to be addressed.”
As a step toward addressing the talent issue, Dr. Nazha developed a course called “No Code – Low Code Machine Learning For Healthcare,” which is available at no cost on AI4healthcare.org.
Even if someone is qualified to build an AI model, a more important task is to identify the right clinical question to be answered by the model.
“I’ve seen multiple models built where the answer to the question we’re trying to answer is useless, clinically not meaningful,” Dr. Nazha said. “Because you can build a model, you can publish it, but nobody can use it, or nobody can derive benefit from it.”
This point about the primacy of problems over models was echoed by Dr. Shouval, who in addition to his medical degree holds a doctoral degree in computer science.
“I never think first of the algorithm and then the problem; I always have a clinical problem in mind, and then I try to apply the best tool,” he said. “Sometimes it’s machine learning, sometimes it’s not. I always look at machine learning just as another tool, just as a means to get to a certain aim and not as a goal in itself.”
The opaqueness of machine learning models is also a major limitation, Dr. Shouval said.
“A lot of [models] are what we call black-box models, where we don’t understand the rationale behind a certain prediction or diagnosis,” he said. “It may be important in medicine for sure, and sometimes for diagnosis, [but] we need to understand what the rationale is, and sometimes it helps us to criticize the prediction or classification.”
Elevating Patient Voices
At the 64th American Society of Hematology Annual Meeting and Exposition, a study was presented that used natural language processing (NLP) and machine learning to shed light on the needs, anxieties, and general sentiments of patients with multiple myeloma (MM) and their caregivers.5
“We do a lot of real-world research to understand patients’ unmet needs and how they are doing after receiving a treatment,” said Dee Lin, PharmD, an Associate Director in the Department of Real World Value & Evidence, Oncology, at Janssen Scientific Affairs, LLC, who was the lead author on the study.
NLP is a subfield of AI that has been around since the 1950s. Today, it is used to extract meanings, relationships, sentiments, and other insights from the vast amounts of free-form text available online or in private databases.
Even though NLP is old school by AI standards, it has proved useful for analyzing the text we all generate when we turn to the internet to connect with others, especially when we are sick. These online conversations on blogs, social media, and patient forums provide valuable real-world data for clinicians and researchers, immersing them in the lives of patients and their caregivers.
Lin cited a number of advantages of this kind of empirical text-mining over traditional methodologies.
“When we think about traditional patient surveys or interviews, [they’re] often guided and highly structured by the researchers, who already have research questions in mind,” she said. “A lot of times patient voices captured in the survey could have been narrowed down [or] filtered by the research questions. And then there are other biases, like the responses are not spontaneous, or there might be recall bias, response bias, and selection bias.”
Social media and patient forum data, in contrast, are unstructured, organic, spontaneous, and more focused on what patients are interested in instead of what researchers are interested in. “It’s more organic and patient initiated,” Lin said.
In the study, the researchers analyzed close to 20,000 posts where patients and their caregivers openly discussed their experiences. They used machine learning tools to run analytics on any tags in the data and to supplement their list of prespecified keywords with alternative synonyms and misspellings to ensure that all relevant posts were captured. They then used NLP tools to classify the posts into specific categories based on topic, sentiment, emotion, and other attributes.
From this research, Lin and colleagues identified a number of areas of unmet patient needs, including access to new treatment options, financial support, living conditions, and caregiver burden.
Patients have varying unmet needs and concerns depending on where they are in their disease journey, Lin explained. Early-stage patients with MM often care more about the risk of side effects, choosing among treatment options, and impacts on quality of life, while those further along may focus more on duration of response and lack of treatment options.
One interesting takeaway from the study was the fact that there were zero mentions of bispecific antibodies in the patient and caregiver posts.
“At the time of the study (May 2020 to June 2022), there was no [US Food and Drug Administration (FDA)]-approved bispecific therapy in the market, so it’s understandable if the physicians don’t talk about it,” she explained. “However, not finding any discussion online potentially indicates there might be a lack of awareness of new therapies in development, so there are definitely some education opportunities there.”
Since the study concluded, the FDA has issued approvals to the company’s two recent bispecific antibodies, teclistamab and talquetamab, for the treatment of relapsed or refractory MM.6,7
Lin pointed out that current value frameworks for medical therapies tend to be focused heavily on efficacy, safety, and cost, but research like hers may help bring patient needs and experiences to the table for a more comprehensive evaluation of the therapies.
“In the current value framework of oncology therapies, patient voices are not sufficiently represented. One of the reasons being it’s just in general difficult to quantify,” Lin explained. “But now, with new technology and the advancement in data, we can use these innovative methods and data sources to create a domain for patient voices and drive the value of innovative therapy beyond those traditional outcomes, which will really help us elevate patient-centered care.”
Lend Me Your Ear, ChatGPT
Patients have been turning to the web for medical self-education, for better or worse, for more than 25 years.8 What is new today, however, is the use of LLM chatbots to access medical information, a development that raises questions about the quality and accuracy of the information.
In a research letter published in JAMA Oncology in August 2023, scientists described how they asked ChatGPT questions about treatments for breast, lung, and prostate cancer and then evaluated the quality of its answers.9 Specifically, three board-certified oncologists reviewed each of the chatbot’s answers and compared them against the recommendations in the National Comprehensive Cancer Network (NCCN) guidelines from 2021.
The good news is that ChatGPT proffered at least one of the gold-standard NCCN recommendations for 102 of 104 (98%) prompts. More worryingly, however, one-third of treatments recommended by the chatbot were at least partially nonconcordant with NCCN guidelines, and 13 of 104 (12.5%) outputs contained “hallucinations” (ie, therapies that were not part of any recommended treatment).
The senior author on the paper, Danielle Bitterman, MD, a radiation oncologist and NLP researcher at the Dana-Farber Cancer Institute/Brigham and Women’s Hospital and Harvard Medical School, said that the results were to be expected.
“It was disappointing that [ChatGPT] included so many wrong recommendations, but we didn’t expect it to meet the high bar of providing clinical recommendations,” Dr. Bitterman said. “The challenge lies in the way it provides information. Unless you have a deep understanding of the subject or access to medical guidelines, it’s challenging to distinguish between the right and wrong responses. This is a feature of the fact that it’s a chatbot, trained to provide responses that sound fluent and make superficial sense.”
Dr. Bitterman also noted that the chatbot generated different responses to slightly reworded questions, explaining “that’s also a challenge that needs to be addressed before [chatbots] are ready to be a reliable source of information for people.”
In a nutshell, chatbots are not yet reliable for medical advice, and people should not seek medical care based on them, said Shan Chen, a doctoral candidate in the Artificial Intelligence in Medicine Program at Harvard Medical School and the first of author of the study.
“There’s a lot of potential behind it, but there’s a lot of work to do to make sure it’s actually safe in really sensitive settings like legal or medical settings,” he said.
Chen said that he had been following the progress of language models for some time, but the release of ChatGPT-3 really changed the game because it made these models widely accessible to people who seem to enjoy chatting with the chatbots.
“There’s a study published from the University of California, San Diego,10 which suggests these bots are more empathetic, which is another quality that is really hard to measure,” he said. “But they’re not factual—that’s a problem.”
On the flip side, using chatbots in health care to manage inbox messages can alleviate doctors’ burnout and stress.11 Chatbots can help sort messages and speed up reply times for critical messages, making health care communication faster, more streamlined, and possibly more empathetic.
“Building a really safe chatbot for the health care system will be an interesting direction,” Chen said.
The Future: LLMs for Drug Discovery?
The usefulness of LLMs like ChatGPT extends beyond their abilities to ease burnout among clinicians or provide patients with an empathetic ear; the technology is positioned to speed up drug discovery and development.12
“Today, to take a drug from target to phase one, it’s about five years on average,” Dr. Nazha explained. “Then to take it from phase one to approval, which is what we call IND to NDA, that’s a minimum [of] five to seven years too. Total, you are talking about 10 years. If you shorten that to about seven years that’s a long time in drug development.”
Dr. Shouval predicts that the technology behind ChatGPT will be harnessed to generate or develop drugs or compounds, or novel combinations thereof, based on analogizing from previous experience.
“You could tell ChatGPT this agent is effective against target A, how about target B?” Dr. Shouval said. “You learn different compounds, different chemical structures, what works and what doesn’t, and then develop new interventions or drugs based on that. This is one of the major promises I see with LLM models like ChatGPT. But again, this is going to require a huge amount of data to train and create useful models.”
He added that this type of technology is “changing the world now, and it’s going to change the way we practice.”
Despite existing limitations and what may be perceived as disappointments, Dr. Bitterman sees a promising future for AI in the field.
“I’m very optimistic about the future of AI and in health care,” Dr. Bitterman said. “I think there’s incredible potential for AI to help people manage information and create new insights. We can start using all the data we’ve been collecting on patients for so long to improve people’s health.”
Leah Sherwood is the Managing Editor for Blood Cancers Today.
References
- Walter W, Pohlkamp C, Meggendorfer M, et al. Artificial intelligence in hematological diagnostics: game changer or gadget? Blood Rev. 2023. doi:10.1016/j.blre.2022.101019
- El Alaoui Y, Elomri A, Qaraqe M, et al. A review of artificial intelligence applications in hematology management: current practices and future prospects. J Med Internet Res. 2022. doi:10.2196/36490
- Chin Neoh S, Srisukkham W, Zhang L, et al. An intelligent decision support system for leukaemia diagnosis using microscopic blood images. Sci Rep. 2015;5:14938. doi:10.1038/srep14938
- Nazha A, Komrokji R, Meggendorfer M, et al. Personalized prediction model to risk stratify patients with myelodysplastic syndromes. J Clin Oncol. 2021;39(33):3737-3746. doi:10.1200/JCO.20.02810
- Lin D, Richardson J, Kim N, et al. Patients’ and caregivers’ perspectives from social media towards disease burden and innovative treatment options in multiple myeloma. Presented at the 64th American Society of Hematology Annual Meeting and Exposition; December 10-13, 2022; New Orleans, Louisiana.
- FDA approves teclistamab-cqyv for relapsed or refractory multiple myeloma. FDA. October 25, 2022. Accessed October 8, 2023. https://www.fda.gov/drugs/resources-information-approved-drugs/fda-approves-teclistamab-cqyv-relapsed-or-refractory-multiple-myeloma
- U.S. FDA approves TALVEY™ (talquetamab-tgvs), a first-in-class bispecific therapy for the treatment of patients with heavily pretreated multiple myeloma. Cision PR Newswire. August 10, 2023. Accessed October 8, 2023. https://www.prnewswire.com/news-releases/us-fda-approves-talvey-talquetamab-tgvs-a-first-in-class-bispecific-therapy-for-the-treatment-of-patients-with-heavily-pretreated-multiple-myeloma-301897786.html
- Jadad AR, Gagliardi A. Rating health information on the internet: navigating to knowledge or to Babel? JAMA. 1998;279(8):611-614. doi:10.1001/jama.279.8.611
- Chen S, Kann BH, Foote MB, et al. Use of artificial intelligence chatbots for cancer treatment information. JAMA Oncol. 2023. doi:10.1001/jamaoncol.2023.2954
- Ayers JW, Poliak A, Dredze M, et al. Comparing physician and artificial intelligence chatbot responses to patient questions posted to a public social media forum. JAMA Intern Med. 2023;183(6):589-596. doi:10.1001/jamainternmed.2023.1838
- Matulis J, McCoy R. Relief in sight? Chatbots, in-baskets, and the overwhelmed primary care clinician. J Gen Intern Med. 2023;38(12):2808-2815. doi:10.1007/s11606-023-08271-8
- Swalla T. AI’s shot in the arm of science and the concerns we need to address. Pharmacy Times. August 15, 2023. Accessed October 8, 2023. https://www.pharmacytimes.com/view/ai-s-shot-in-the-arm-of-science-and-the-concerns-we-need-to-address