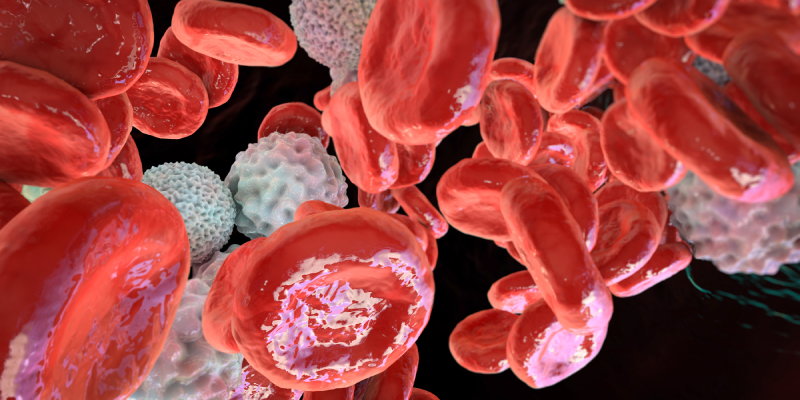
A machine learning tool showcased at the 65th ASH Annual Meeting and Exposition could help identify the relatively small number of polycythemia vera (PV) patients vulnerable to thrombosis. Knowing who is at high risk in the near term could make it easier to conduct trials of anti-thrombotic therapies, investigators said.
With available treatments, the annual risk for thrombosis in PV is as low as 1% to 5% per year, making it a challenge to design trials of anti-thrombotic therapies that are large and long enough to show an improvement in thrombosis-free survival, noted Spencer Krichevsky, a biomedical informatics PhD student at Stonybrook University in New York, and colleagues. Currently, European LeukemiaNet (ELN) criteria may be used to select patients at high risk for thrombosis to enroll in trials, but Krichevsky’s team sees room to improve efficiency using machine learning.
For the study, the researchers trained more than a million models on a large volume of clinical data, including age, time since PV diagnosis, and changes in body mass index. They gathered data for 526 patients from electronic health records and a research database and used the data for three purposes: model training, testing, and validation.
Krichevsky and colleagues reported that the machine learning model was very good at predicting thrombosis risk (F1=0.91, area under the curve (AUC)=0.84) compared with ELN (F1=0.1, AUC=0.39). In practical terms, the data suggest that to show a 50% reduction in thrombosis risk in a trial, it would take 1,000 patients using ELN risk criteria versus only 80 with the machine learning method, researchers pointed out. Further testing and validation are now ongoing.
Reference
Krichevsky S, Silver R, Abu-Zeinah G, et al. Using machine learning to predict near-term thrombosis risk in patients with polycythemia vera. Abstract #3186. Presented at the 65th ASH Annual Meeting and Exposition; December 9-12; San Diego, California.