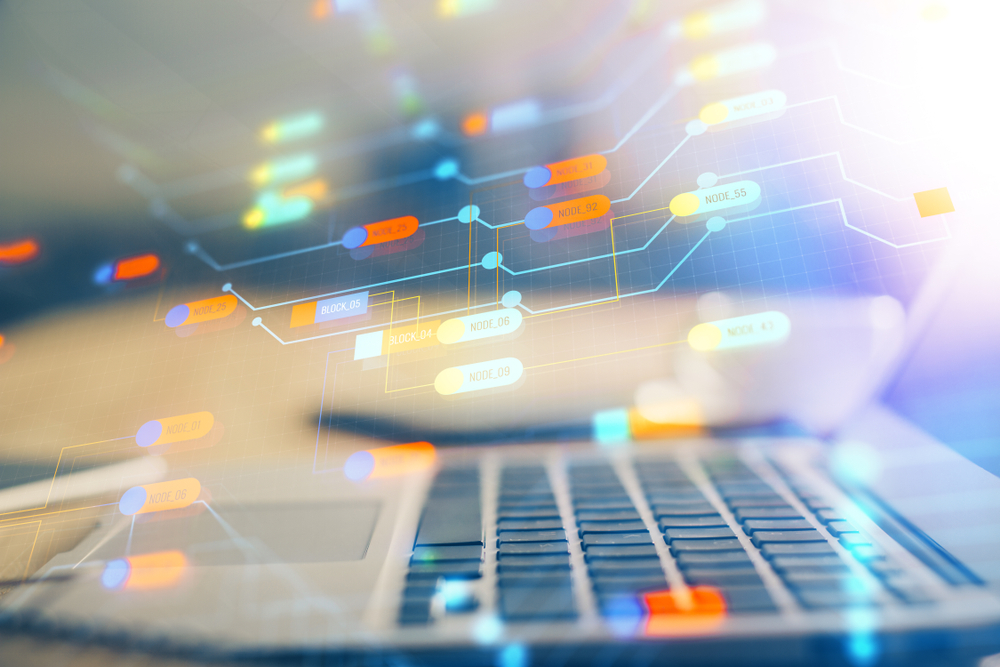
Researchers have developed and validated deep learning models to aid in the diagnosis of myeloproliferative neoplasms (MPN) and to help with subtype differentiation. Zhongxun Shi, of Jiangsu Province Hospital, Nanjing, China, and colleagues presented the details of these models at the 65th American Society of Hematology Annual Meeting & Exposition.
According to the abstract, accurate diagnosis of MPN can be hampered by the subjectivity of morphological assessment and overlapping pathological features of different MDS subtypes. Therefore, the researchers developed clinical, deep learning, and fusion diagnosis models using clinical parameters, a whole slide images–based deep learning algorithm using hematoxylin-eosin staining of bone marrow specimens, and a combination of both.
They enrolled 1,051 patients with MPN and divided them into training, internal testing, internal validation, and external validation cohorts. In the combined validation cohort, the researchers found that the clinical model performed best for polycythemia vera (PV) and the fusion model may have clinical utility in identifying both essential thrombocytopenia (ET) and prefibrotic/early primary myelofibrosis (pre-PMF). The fusion model performed best in distinguishing MPN from non-MPN controls and had the best performance in the identification of ET and pre-PMF by reducing the number of misclassified pre-PMF and the number of ET cases misclassified as pre-PMF.
The researchers also compared their deep learning models with three junior hematopathologists with less than five years of clinical experience and three senior hematopathologists with more than 10 years of experience. One hundred cases were randomly selected from the pool of validation sets, and the hematopathologists were blinded to the MPN label.
The clinical model performed best for PV; its performance was equivalent to the senior hematopathologists and higher than the junior hematopathologists. For ET and pre-PMF, the fusion model performed better than junior hematopathologists and comparable to senior hematopathologists.
Reference
Wang R, Shi Z, Zhang Y, et al. Development and validation of deep learning model for diagnosis and subtypes differentiation of myeloproliferative neoplasms using clinical data and digital pathology. Abstract #123. Presented at the 65th ASH Annual Meeting and Exposition; December 9-12, 2023; San Diego, California.